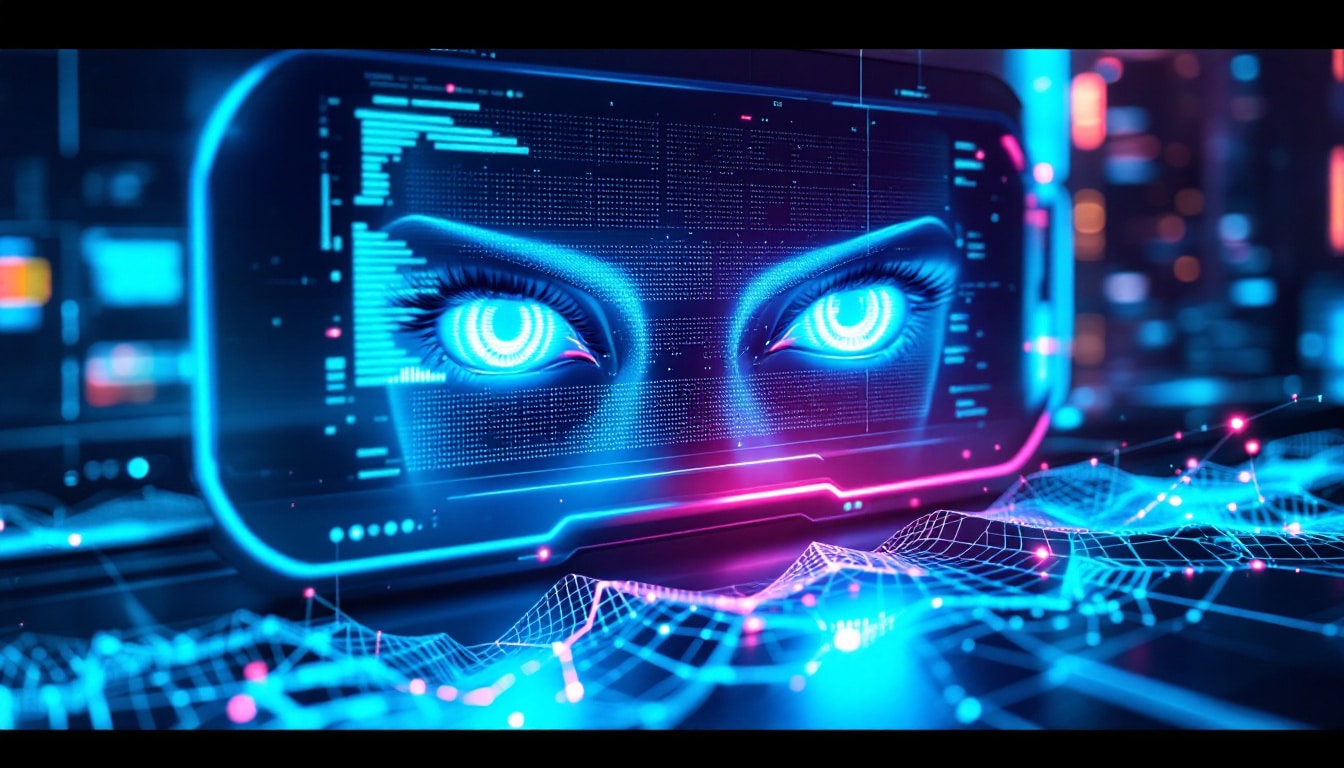
The financial markets have witnessed a revolutionary transformation through artificial intelligence technology. Modern crypto trading bots and AI-powered systems have fundamentally changed how traders interact with markets, analyze data, and execute trades.
The integration of machine learning algorithms and advanced data analytics has enabled these systems to process vast amounts of market information in real-time, identifying patterns and opportunities that human traders might miss.
Recent studies show that AI-powered trading systems can analyze over 1 million data points per second, compared to a human trader’s capacity of processing only a few dozen. This advancement has also simplified the process to buy XRP and other cryptocurrency, democratizing sophisticated trading strategies and making them accessible to both institutional and retail investors across global markets.
Early Years of Algorithmic Trading
The history of algorithmic trading traces back through centuries, with early systematic approaches emerging during historic market events. The true breakthrough came in the late 1960s with the New York Stock Exchange’s DOT (designated order turnaround) system.
This innovation marked the first step toward automated trading infrastructure, enabling faster and more efficient communication between traders and floor specialists. The development of DOT represented a crucial shift from manual to electronic order processing, reducing execution times from minutes to seconds.
During the 1970s, the system handled an average of 100,000 orders per day, a volume that would have been impossible to process manually. The implementation of DOT also led to a 40% reduction in trading errors and a significant decrease in transaction costs.
The DOT system, later upgraded to SuperDOT, functioned as a primitive API for trading operations. By the mid-1980s, SuperDOT was processing over 80% of all NYSE orders.
The 1980s saw further advancement with the introduction of algorithm-controlled risk management systems, particularly in the derivatives markets. Portfolio insurance strategies, based on the Black-Scholes model, became increasingly popular, with total assets under management reaching $60 billion by 1987.
These early systems demonstrated the potential for automated decision-making in financial markets, setting the stage for more sophisticated trading algorithms.
Significant Changes in the 90s
The 1990s heralded a new era with the emergence of Electronic Communication Networks (ECNs). These interconnected computer systems revolutionized stock trading by making markets more accessible to outside participants. The introduction of ECNs led to a 65% reduction in trading costs and increased daily trading volume by 300% between 1995 and 2000.
The pivotal development in 2001 when the tick size was reduced to $0.01 enabled microtrading strategies and more sophisticated automation, resulting in an explosion of algorithmic trading activity.
This period also marked the rise of AI-driven trading systems, with IBM’s MGD and HP’s ZIP platforms demonstrating remarkable success. In controlled testing environments, these systems achieved win rates of up to 75% against human traders, with reaction times averaging 100 milliseconds compared to a human’s 150-300 milliseconds.
The success of these early AI systems sparked a technological arms race among financial institutions, leading to billions in investment in automated trading technology.
Market Structure Evolution
The transformation of trading ecosystems accelerated with the digitization of forex markets and other financial instruments. Daily forex trading volume increased from $1.2 trillion in 1995 to over $6.6 trillion by 2019, largely facilitated by electronic trading systems.
This evolution prompted the SEC to implement the Regulation National Market System, enforcing the “trade through rule” to ensure optimal pricing for all market participants.
The new framework created a structured environment for algorithmic trading while maintaining market integrity. Implementation of Reg NMS led to a 45% improvement in price discovery and reduced spreads by an average of 35% across major exchanges.
The regulatory changes coincided with technological improvements in trading infrastructure. Average trade execution times dropped from 250 milliseconds to under 100 microseconds between 2004 and 2010.
These developments enabled complex event processing capabilities, with systems able to monitor and react to over 100,000 market events per second. Dark pools and alternative trading systems emerged, accounting for approximately 40% of all US equity trading volume by 2015.
Rise of Institutional Algorithms
Major financial institutions developed increasingly sophisticated proprietary trading algorithms. BNP Paribas’s Chameleon and Credit Suisse’s Sniper and Guerilla systems revolutionized institutional trading.
These platforms enabled complex strategies like statistical arbitrage and mean reversion, processing market data at unprecedented speeds. By 2012, algorithmic trading systems could execute trades in under 10 microseconds, compared to 20-30 milliseconds in the early 2000s.
High-frequency trading dominated markets by 2009, accounting for 60% of equity trading volume and generating estimated annual profits of $5 billion. The forex market saw even higher adoption rates, with automated trading systems handling over 80% of all orders.
Investment in trading technology reached $1.5 billion annually among top-tier banks, with algorithm development teams growing from dozens to hundreds of specialists.
The Era of AI Cryptocurrency Trading
By 2015, the financial landscape transformed with cryptocurrency markets. Major institutions like J.P. Morgan and Fidelity Investment incorporated Bitcoin into their portfolios, with institutional cryptocurrency holdings reaching $6.5 billion by 2020.
Advanced trading platforms emerged, offering automated solutions that could analyze over 500 cryptocurrency pairs simultaneously while executing multiple strategies.
Companies like WunderTrading pioneered features including DCA buying, GRID bots, and statistical arbitrage systems. These platforms processed over 1 million transactions daily, with success rates exceeding 65% in volatile market conditions.
Machine learning algorithms improved trading performance by an average of 25% compared to traditional technical analysis approaches. The cryptocurrency market’s 24/7 nature made automated trading systems essential, with bots handling over 75% of total trading volume on major exchanges.
Benefits of AI Trading Bots
AI trading systems offer several advantages over traditional methods. Operating without emotional bias, these systems maintain consistent performance regardless of market conditions, reducing human error by up to 80%. The ability to function 24/7 enables exploitation of opportunities across time zones, with some systems managing to identify and execute profitable trades within milliseconds.
The continuous learning capability of AI systems represents another crucial advantage. Modern trading algorithms can analyze historical data spanning decades, identifying patterns with 90% accuracy.
These platforms adapt to evolving market conditions, with neural networks processing millions of data points to optimize trading strategies. Studies show AI-powered systems outperform traditional trading approaches by 15-30% on average, while reducing operational costs by up to 50%.
Future Implications and Conclusion
The integration of AI in trading represents a fundamental shift in financial markets. The democratization of sophisticated tools continues to level the playing field, with retail traders now accessing capabilities previously exclusive to institutions. Market data shows that AI-powered trading systems now manage over $2 trillion in assets globally, highlighting their growing importance.
As AI technology evolves, its role will become more central to trading success. Next-generation systems incorporating quantum computing and advanced neural networks promise even greater capabilities, with potential improvements in prediction accuracy exceeding 40%. The future of trading lies in strategically combining human insight with AI capabilities, creating a new paradigm in financial markets.